
Bayesian Survival Analysis
By:Â Joseph G. Ibrahim, Ming-Hui Chen, Debajyoti Sinha
Hardcover | 7 December 2004
At a Glance
504 Pages
23.39 x 15.6 x 2.69
Hardcover
$317.33
or 4 interest-free payments of $79.33 with
 orÂAims to ship in 7 to 10 business days
Industry Reviews
From the reviews:
"The analysis of time-event data arises naturally in many fields of study. This book focuses exclusively on medicine and public health but the methods presented can be applied in a number of other areas, including biology, economics and engineering. Although several previously published texts address survival analysis from a frequentist perspective, this book examines solely Bayesian approaches to survival analysis. Recent advances in computing and practical methods for prior elicitation have now made Bayesian survival analysis of complex models feasible. This book provides a comprehensive and modern treatment of the subject. In addition, the authors demonstrate the use of the statistical package BUGS for several of the models and methodologies discussed in the book. The authors provide a collection of theoretical and applied problems in the exercises at the end of each chapter."
ISI Short Book Reviews, April 2002
"This is definitely a worthwhile read for any statistician specializing in survival analysis. It is pitched so that part of it is readily usable by the medical statisitciann, but it will also provide stimulation for statisticians involved in methodological development or the writing of new software for survival analysis." International Journal of Epidemiology
"Many books have been published concerning survival analysis or Bayesian methods; Bayesian Survival Analysis is the first comprehensive treatment that combines these two important areas of statistics. Ibrahim, Chen, and Sinha have made an admirable accomplishment on the subject in a well-organized and easily accessible fashion." Journal of the American Statistical Association
"This is one of the best combinations of advanced methodology and practical applications that I have ever encountered." Technometrics, May 2002
"This is a book by three authors who are well-known for their contribution to Bayesian survival analysis. ... It is a good book with many areas of strength. ... There are several new methods, ideas, results, some of which are due to the authors. There is a good discussion of historical priors ... . Other things that strike me as new are a good technical discussion of frailty and cure models ... . I have learnt a lot and enjoyed reading the book." (Jayanta K. Ghosh, Sankhya: The Indian Journal of Statistics, Vol. 65 (3), 2003)
"This book illustrates several Bayesian techniques to analyze survival data in biology, medicine, public health, epidemiology, clinical trials, and economics. ... It could be used as a textbook in a graduate level course. ... In particular, I enjoyed the presentations of cure models and cancer vaccine trials. Biostatisticians will like reading this book from the Bayesian points of view." (Ramalingam Shanmugam, Journal of Statistical Computation and Simulation, Vol. 74 (10), 2004)
"This book offers an excellent and thorough summary of an exciting methodological development since the seventies of the last century. ... The authors offer a gentle journey through the archipelago of Bayesian Survival analysis. They combine in a pleasant way theory, examples, and exercises. ... I hope that this stimulating book may tempt many readers to enter the field of Bayesian survival analysis ... ." (Ulrich Mansmann, Metrika, September, 2004)
"It offers a presentation of Bayesian methods in Survival Analysis that is, at a time, comprehensive and suitably balanced between theory and applications; many relevant models and methods are illustrated and most of them are provided with detailed examples and case studies drawn from the medical research. ... The book offers a quite up-to-date view of Bayesian Statistics and accounts extensively for Monte Carlo-based sampling methods and for the various methods of prior elicitation, suitable to cope with non-parametric as well as with semi-parametric models." (Fabio Spizzichino, Statistics in Medicine, Vol. 23, 2004)
"This is not an elementary book. ... The book develops methodology and does this at a high level, because the reader is presumed to have a mathematical statistics background in both classical and Bayesian methods. Happily, the book is replete with examples. This is one of the best combinations of advanced methodology and practical applications that I have encountered. ... Computing support for the book comes from the package called BUGS ... ." (Technometrics, Vol. 44 (2), 2002)
"This book provides a comprehensive treatment of Bayesian survival analysis. Several topics are addressed, including parametric models, semiparametric models based on prior processes, proportional and non-proportional hazards models, frailty models, cure rate models, model selection and comparison ... . The book presents a balance between theory and applications, and for each class of models discussed, detailed examples and analyses from case studies are presented whenever possible." (L'Enseignement Mathematique, Vol. 48 (1-2), 2002)
"The book is about Bayesian survival analysis which is illustrated with examples that mostly use the BUGS software package. ... this is definitively a worthwhile read for any statistician specializing in survival analysis. It is pitched so that part of it is readily usable by the medical statistician, but it will also provide stimulation for statisticians involved in methodological development or the writing of new software for survival analysis." (Margaret May, International Journal of Epidemiology, Vol. 31 (2), 2002)
"This book focuses exclusively on medicine and public health but the methods presented can be applied in a number of other areas, including biology, economics and engineering. ... This book provides a comprehensive and modern treatment of the subject. In addition, the authors demonstrate the use of the statistical package BUGS for several of the models and methodologies discussed in the book. The authors provide a collection of theoretical and applied problems in the exercises at the end of each chapter." (C. M. O'Brien, Short Book Reviews, Vol. 22 (1), 2002)
"Ibrahim, Chen and Sinha command over a rich experience in both Bayesian and survival analysis. Drawing from this experience they have put together a comprehensive description of Bayesian methodology in survival analysis. The book is written for researchers and graduate students. ... The book is a useful tool for practitioners who analyze survival data using Bayesian methods." (Mathias Schaller, Statistical Papers, Vol. 47, 2005)
Preface | p. vii |
Introduction | p. 1 |
Aims | p. 1 |
Outline | p. 2 |
Motivating Examples | p. 3 |
Survival Analysis | p. 13 |
Proportional Hazards Models | p. 15 |
Censoring | p. 15 |
Partial Likelihood | p. 16 |
The Bayesian Paradigm | p. 17 |
Sampling from the Posterior Distribution | p. 18 |
Informative Prior Elicitation | p. 22 |
Why Bayes? | p. 26 |
Exercises | p. 27 |
Parametric Models | p. 30 |
Exponential Model | p. 30 |
Weibull Model | p. 35 |
Extreme Value Model | p. 37 |
Log-Normal Model | p. 39 |
Gamma Model | p. 40 |
Exercises | p. 42 |
Semiparametric Models | p. 47 |
Piecewise Constant Hazard Model | p. 47 |
Models Using a Gamma Process | p. 50 |
Gamma Process on Cumulative Hazard | p. 50 |
Gamma Process with Grouped-Data Likelihood | p. 51 |
Relationship to Partial Likelihood | p. 53 |
Gamma Process on Baseline Hazard | p. 55 |
Prior Elicitation | p. 56 |
Approximation of the Prior | p. 57 |
Choices of Hyperparameters | p. 59 |
Sampling from the Joint Posterior Distribution of ([beta], [delta], a[subscript 0]) | p. 60 |
A Generalization of the Cox Model | p. 63 |
Beta Process Models | p. 66 |
Beta Process Priors | p. 66 |
Interval Censored Data | p. 71 |
Correlated Gamma Processes | p. 72 |
Dirichlet Process Models | p. 78 |
Dirichlet Process Prior | p. 78 |
Dirichlet Process in Survival Analysis | p. 81 |
Dirichlet Process with Doubly Censored Data | p. 84 |
Mixtures of Dirichlet Process Models | p. 87 |
Conjugate MDP Models | p. 89 |
Nonconjugate MDP Models | p. 90 |
MDP Priors with Censored Data | p. 91 |
Inclusion of Covariates | p. 94 |
Exercises | p. 94 |
Frailty Models | p. 100 |
Proportional Hazards Model with Frailty | p. 101 |
Weibull Model with Gamma Frailties | p. 102 |
Gamma Process Prior for H[subscript 0](t) | p. 104 |
Piecewise Exponential Model for h[subscript 0](t) | p. 106 |
Positive Stable Frailties | p. 112 |
A Bayesian Model for Institutional Effects | p. 118 |
Posterior Likelihood Methods | p. 126 |
Methods Based on Partial Likelihood | p. 131 |
Multiple Event and Panel Count Data | p. 134 |
Multilevel Multivariate Survival Data | p. 136 |
Bivariate Measures of Dependence | p. 147 |
Exercises | p. 148 |
Cure Rate Models | p. 155 |
Introduction | p. 155 |
Parametric Cure Rate Model | p. 156 |
Models | p. 156 |
Prior and Posterior Distributions | p. 160 |
Posterior Computation | p. 163 |
Semiparametric Cure Rate Model | p. 171 |
An Alternative Semiparametric Cure Rate Model | p. 179 |
Prior Distributions | p. 180 |
Multivariate Cure Rate Models | p. 185 |
Models | p. 185 |
The Likelihood Function | p. 188 |
The Prior and Posterior Distributions | p. 190 |
Computational Implementation | p. 191 |
Appendix | p. 199 |
Exercises | p. 205 |
Model Comparison | p. 208 |
Posterior Model Probabilities | p. 209 |
Variable Selection in the Cox Model | p. 210 |
Prior Distribution on the Model Space | p. 211 |
Computing Prior and Posterior Model Probabilities | p. 212 |
Criterion-Based Methods | p. 219 |
The L Measure | p. 220 |
The Calibration Distribution | p. 223 |
Conditional Predictive Ordinate | p. 227 |
Bayesian Model Averaging | p. 234 |
BMA for Variable Selection in the Cox Model | p. 236 |
Identifying the Models in A' | p. 237 |
Assessment of Predictive Performance | p. 239 |
Bayesian Information Criterion | p. 246 |
Model Selection Using BIC | p. 249 |
Exponential Survival Model | p. 249 |
The Cox Proportional Hazards Model | p. 250 |
Exercises | p. 254 |
Joint Models for Longitudinal and Survival Data | p. 262 |
Introduction | p. 262 |
Joint Modeling in AIDS Studies | p. 263 |
Joint Modeling in Cancer Vaccine Trials | p. 263 |
Joint Modeling in Health-Related Quality of Life Studies | p. 264 |
Methods for Joint Modeling of Longitudinal and Survival Data | p. 265 |
Partial Likelihood Models | p. 265 |
Joint Likelihood Models | p. 267 |
Mixture Models | p. 273 |
Bayesian Methods for Joint Modeling of Longitudinal and Survival Data | p. 275 |
Exercises | p. 287 |
Missing Covariate Data | p. 290 |
Introduction | p. 290 |
The Cure Rate Model with Missing Covariate Data | p. 292 |
A General Class of Covariate Models | p. 293 |
The Prior and Posterior Distributions | p. 297 |
Model Checking | p. 301 |
Appendix | p. 311 |
Exercises | p. 317 |
Design and Monitoring of Randomized Clinical Trials | p. 320 |
Group Sequential Log-Rank Tests for Survival Data | p. 320 |
Bayesian Approaches | p. 322 |
Range of Equivalence | p. 326 |
Prior Elicitation | p. 328 |
Predictions | p. 332 |
Checking Prior-Data Compatibility | p. 334 |
Bayesian Sample Size Determination | p. 336 |
Alternative Approaches to Sample Size Determination | p. 340 |
Exercises | p. 349 |
Other Topics | p. 352 |
Proportional Hazards Models Built from Monotone Functions | p. 352 |
Likelihood Specification | p. 354 |
Prior Specification | p. 356 |
Time-Dependent Covariates | p. 357 |
Accelerated Failure Time models | p. 359 |
MDP Prior for [theta subscript i] | p. 360 |
Polya Tree Prior for [theta subscript i] | p. 364 |
Bayesian Survival Analysis Using MARS | p. 373 |
The Bayesian Model | p. 374 |
Survival Analysis with Frailties | p. 379 |
Change Point Models | p. 381 |
Basic Assumptions and Model | p. 382 |
Extra Poisson Variation | p. 385 |
Lag Functions | p. 386 |
Recurrent Tumors | p. 388 |
Bayesian Inference | p. 389 |
The Poly-Weibull Model | p. 395 |
Likelihood and Priors | p. 396 |
Sampling the Posterior Distribution | p. 397 |
Flexible Hierarchical Survival Models | p. 398 |
Three Stages of the Hierarchical Model | p. 400 |
Implementation | p. 403 |
Bayesian Model Diagnostics | p. 413 |
Bayesian Latent Residuals | p. 413 |
Prequential Methods | p. 417 |
Future Research Topics | p. 429 |
Appendix | p. 431 |
Exercises | p. 433 |
List of Distributions | p. 436 |
References | p. 438 |
Author Index | p. 467 |
Subject Index | p. 475 |
Table of Contents provided by Rittenhouse. All Rights Reserved. |
ISBN: 9780387952772
ISBN-10: 0387952772
Series: Springer Series in Statistics
Published: 7th December 2004
Format: Hardcover
Language: English
Number of Pages: 504
Audience: Professional and Scholarly
Publisher: Springer Nature B.V.
Country of Publication: US
Dimensions (cm): 23.39 x 15.6 x 2.69
Weight (kg): 0.88
Shipping
Standard Shipping | Express Shipping | |
---|---|---|
Metro postcodes: | $9.99 | $14.95 |
Regional postcodes: | $9.99 | $14.95 |
Rural postcodes: | $9.99 | $14.95 |
How to return your order
At Booktopia, we offer hassle-free returns in accordance with our returns policy. If you wish to return an item, please get in touch with Booktopia Customer Care.
Additional postage charges may be applicable.
Defective items
If there is a problem with any of the items received for your order then the Booktopia Customer Care team is ready to assist you.
For more info please visit our Help Centre.
You Can Find This Book In

Superconvergence
How the Genetics, Biotech, and AI Revolutions Will Transform our Lives, Work, and World
Hardcover
RRP $59.99
$50.90
OFF
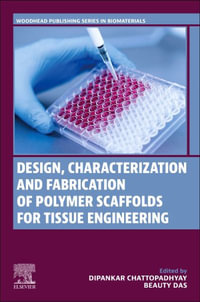
Design, Characterization and Fabrication of Polymer Scaffolds for Tissue Engineering
Woodhead Publishing Biomaterials
Paperback
RRP $405.95
$292.25
OFF

Biofuels and Sustainability
Life-cycle Assessments, System Biology, Policies, and Emerging Technologies
Paperback
RRP $414.95
$298.50
OFF
This product is categorised by
- Non-FictionEngineering & TechnologyBiochemical EngineeringBiotechnology
- Non-FictionMathematicsProbability & Statistics
- Non-FictionScienceScience in GeneralMaths for Scientists
- Non-FictionScienceBiology, Life SciencesLife Sciences in General
- Non-FictionMedicineMedicine in GeneralPublic Health & Preventive MedicineEpidemiology & Medical Statistics