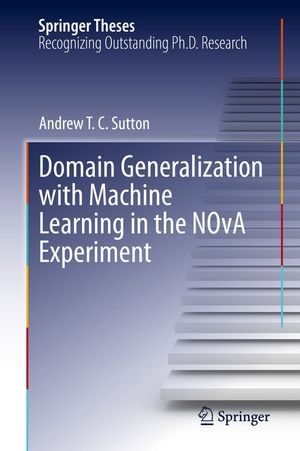
Domain Generalization with Machine Learning in the NOvA Experiment
eText | 8 November 2023
At a Glance
eText
$249.00
or
Instant online reading in your Booktopia eTextbook Library *
Read online on
Desktop
Tablet
Mobile
Not downloadable to your eReader or an app
Why choose an eTextbook?
Instant Access *
Purchase and read your book immediately
Read Aloud
Listen and follow along as Bookshelf reads to you
Study Tools
Built-in study tools like highlights and more
* eTextbooks are not downloadable to your eReader or an app and can be accessed via web browsers only. You must be connected to the internet and have no technical issues with your device or browser that could prevent the eTextbook from operating.
ISBN: 9783031435836
ISBN-10: 3031435834
Series: Springer Theses
Published: 8th November 2023
Format: ePUB
Language: English
Publisher: Springer Nature
You Can Find This eBook In
This product is categorised by
- Non-FictionSciencePhysicsNuclear Physics
- Non-FictionSciencePhysicsParticle & High-Energy Physics
- Non-FictionEngineering & TechnologyTechnology in GeneralInstruments & Instrumentation EngineeringEngineering Measurement & Calibration
- Non-FictionScienceScience in GeneralScientific StandardsMensuration & Systems of Measurement
- Non-FictionComputing & I.T.Computer ScienceArtificial IntelligenceMachine Learning
- Non-FictionMathematicsProbability & Statistics