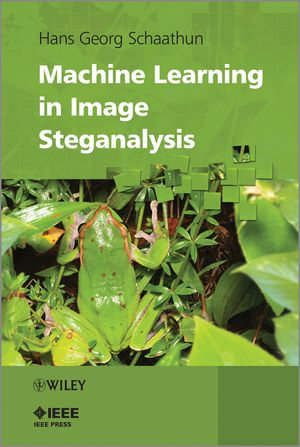
eBOOK
Machine Learning in Image Steganalysis
eBook | 18 May 2016 | Edition Number 1
At a Glance
296 Pages
eBook
$149.99
or 4 interest-free payments of $37.50 with
orInstant Digital Delivery to your Booktopia Reader App
Read on
Steganography is the art of communicating a secret message, hiding the very existence of a secret message. This book is an introduction to steganalysis as part of the wider trend of multimedia forensics, as well as a practical tutorial on machine learning in this context. It looks at a wide range of feature vectors proposed for steganalysis with performance tests and comparisons. Python programs and algorithms are provided to allow readers to modify and reproduce outcomes discussed in the book.
Read on
Preface xi
PART I OVERVIEW
1 Introduction 3
1.1 Real Threat or Hype? 3
1.2 Artificial Intelligence and Learning 4
1.3 How to Read this Book 5
2 Steganography and Steganalysis 7
2.1 Cryptography versus Steganography 7
2.2 Steganography 8
2.3 Steganalysis 17
2.4 Summary and Notes 23
3 Getting Started with a Classifier 25
3.1 Classification 25
3.2 Estimation and Confidence 28
3.3 Using libSVM 30
3.4 Using Python 33
3.5 Images for Testing 38
3.6 Further Reading 39
PART II FEATURES
4 Histogram Analysis 43
4.1 Early Histogram Analysis 43
4.2 Notation 44
4.3 Additive Independent Noise 44
4.4 Multi-dimensional Histograms 54
4.5 Experiment and Comparison 63
5 Bit-plane Analysis 65
5.1 Visual Steganalysis 65
5.2 Autocorrelation Features 67
5.3 Binary Similarity Measures 69
5.4 Evaluation and Comparison 72
6 More Spatial Domain Features 75
6.1 The Difference Matrix 75
6.2 Image Quality Measures 82
6.3 Colour Images 86
6.4 Experiment and Comparison 86
7 The Wavelets Domain 89
7.1 A Visual View 89
7.2 The Wavelet Domain 90
7.3 Farid's Features 96
7.4 HCF in the Wavelet Domain 98
7.5 Denoising and the WAM Features 101
7.6 Experiment and Comparison 106
8 Steganalysis in the JPEG Domain 107
8.1 JPEG Compression 107
8.2 Histogram Analysis 114
8.3 Blockiness 122
8.4 Markov Model-based Features 124
8.5 Conditional Probabilities 126
8.6 Experiment and Comparison 128
9 Calibration Techniques 131
9.1 Calibrated Features 131
9.2 JPEG Calibration 133
9.3 Calibration by Downsampling 137
9.4 Calibration in General 146
9.5 Progressive Randomisation 148
PART III CLASSIFIERS
10 Simulation and Evaluation 153
10.1 Estimation and Simulation 153
10.2 Scalar Measures 158
10.3 The Receiver Operating Curve 161
10.4 Experimental Methodology 170
10.5 Comparison and Hypothesis Testing 173
10.6 Summary 176
11 Support Vector Machines 179
11.1 Linear Classifiers 179
11.2 The Kernel Function 186
11.3 ?-SVM 189
11.4 Multi-class Methods 191
11.5 One-class Methods 192
11.6 Summary 196
12 Other Classification Algorithms 197
12.1 Bayesian Classifiers 198
12.2 Estimating Probability Distributions 203
12.3 Multivariate Regression Analysis 209
12.4 Unsupervised Learning 212
12.5 Summary 215
13 Feature Selection and Evaluation 217
13.1 Overfitting and Underfitting 217
13.2 Scalar Feature Selection 220
13.3 Feature Subset Selection 222
13.4 Selection Using Information Theory 225
13.5 Boosting Feature Selection 238
13.6 Applications in Steganalysis 239
14 The Steganalysis Problem 245
14.1 Different Use Cases 245
14.2 Images and Training Sets 250
14.3 Composite Classifier Systems 258
14.4 Summary 262
15 Future of the Field 263
15.1 Image Forensics 263
15.2 Conclusions and Notes 265
Bibliography 267
Index 279
ISBN: 9781118437988
ISBN-10: 1118437985
Series: IEEE Press
Published: 18th May 2016
Format: ePUB
Language: English
Number of Pages: 296
Audience: Professional and Scholarly
Publisher: Wiley
Country of Publication: GB
Edition Number: 1